First article in a series covering scraping data from the web into R; Part II (scraping JSON data) is here, Part III (targeting data using CSS selectors) is here, and we give some suggestions on potential projects here. Video downloading apps for mac.
There is a massive amount of data available on the web. Some of it is in the form of formatted, downloadable data-sets which are easy to access. But the majority of online data exists as web content such as blogs, news stories and cooking recipes. With formatted files, accessing the data is fairly straightforward; just download the file, unzip if necessary, and import into R.
Web scraping for price comparison. As the market wisdom says, price is everything. Torrent civilization beyond earth for mac os. Welcome to Web Scraping in R. After watching this video, you will be able to identify the components of an HTML page and then perform common web scraping tasks, like reading, downloading, and extracting data from a web page, using the rvest package in R. HTML stands for Hypertext Markup Language and it is used mainly for writing web pages.
For “wild” data however, getting the data into an analyzable format is more difficult. Accessing online data of this sort is sometimes referred to as “web scraping”. You will need to download the target page from the Internet and extract the information you need. Two R facilities, readLines()
from the base package and getURL()
from the RCurl package make this task possible.
readLines
For basic web scraping tasks the readLines()
function will usually suffice. readLines()
allows simple access to webpage source data on non-secure servers. In its simplest form, readLines()
takes a single argument – the URL of the web page to be read:
As an example of a (somewhat) practical use of web scraping, imagine a scenario in which we wanted to know the 10 most frequent posters to the R-help listserve for January 2009. Because the listserve is on a secure site (e.g. it has https:// rather than http:// in the URL) we can’t easily access the live version with readLines()
. So for this example, I’ve posted a local copy of the list archives on the this site.
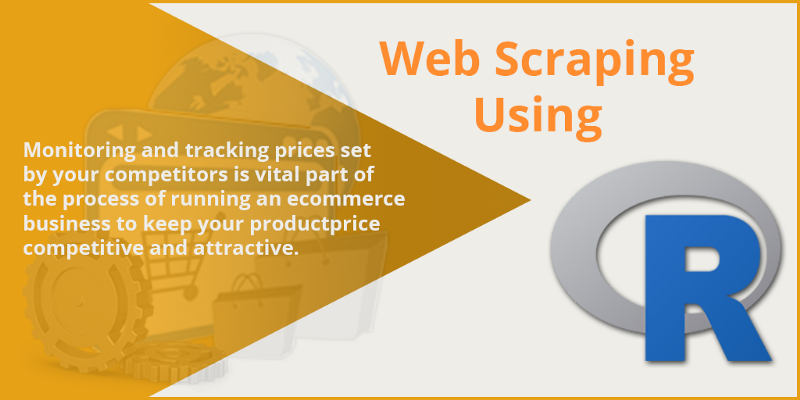
One note, by itself readLines()
can only acquire the data. You’ll need to use grep(), gsub()
or equivalents to parse the data and keep what you need. A key challenge in web scraping is finding a way to unpack the data you want from a web page full of other elements.
We can see that Gabor Grothendieck was the most frequent poster to R-help in January 2009.

Looking Under The Hood
To understand why this example was so straightforward, here is a closer look at the underlying HTML:
Honestly, this is about as user friendly as you can get with HTML data formatted “in the wild”. The data element we are interested in (poster name) is broken out as the main element on its own line. We can quickly and easily grab these lines using grep()
. Once we have the lines we’re interested in, we can trim them down by using gsub()
to replace the unwanted HTML code.
Incidentally, for those of you who are also web developers, this can be a huge time saver for repetitive tasks. If you’re not working with anything highly sensitive, add a few simple “data dump” pages to your site and use readLines()
to pull back the data when you need it. This is great for progress reporting and status updates. Just be sure to keep page design simple – basic, well formatted HTML with minimal fluff.
Looking for A Test Project? Check Out our Big List of Web Scraping Project Ideas!
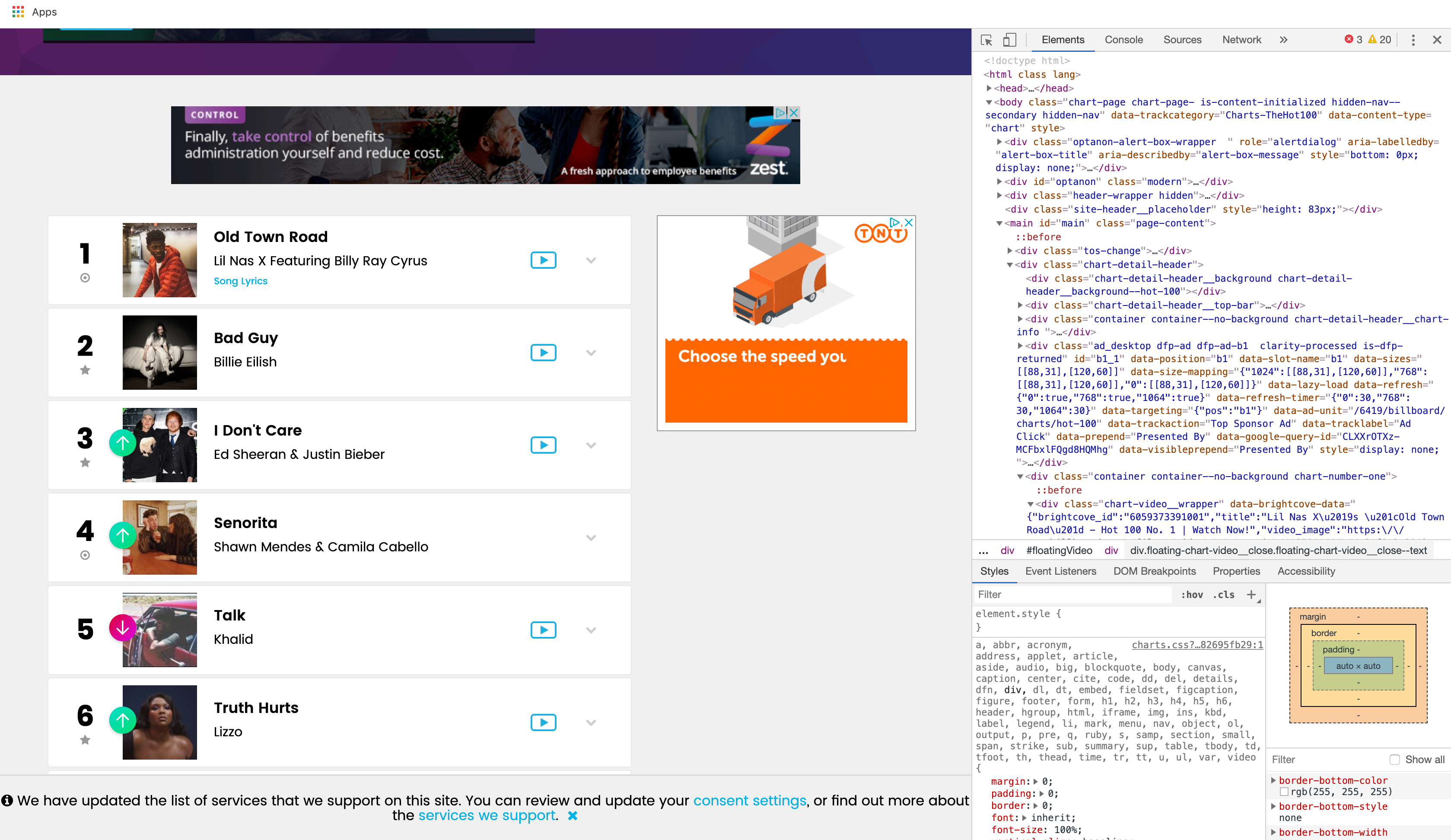
The RCurl package
To get more advanced http features such as POST capabilities and https access, you’ll need to use the RCurl package. To do web scraping tasks with the RCurl package use the getURL()
function. After the data has been acquired via getURL()
, it needs to be restructured and parsed. The htmlTreeParse()
function from the XML package is tailored for just this task. Using getURL()
we can access a secure site so we can use the live site as an example this time.
For basic web scraping tasks readLines()
will be enough and avoids over complicating the task. For more difficult procedures or for tasks requiring other http features getURL()
or other functions from the RCurl package may be required.
This was the first in our series on web scraping. Check out one of the later articles to learn more about scraping:
- Scraper Ergo Sum – Suggested projects for going deeper on web scraping
You may also be interested in the following
Webscraping in R
Using what was covered in the lectures, write a program in R to collect data via webscraping.
The website the data is collected from must allow webscraping. There are numerous websites that offer directions on how to webscrape. Have you visited any of these sources? How many mention legality?
After scraping data from a site write a research paper to describe:
The data collected, how you chose this data and how legality was confirmed
What issues you may have ran into in the data collection
How you may use webscraping in a practical setting, such as research or for an employer
Discuss the legality of webscraping outside the scope of this data, what problems can webscraping cause?
The following documents should be submitted for full credit:
The research paper
The .r file with your webscraping code
Free Web Scraper
Your research paper should be at least 3 pages (and at least 800 words), double-spaced, saved in MS Word format. All research papers in this course should be written in APA format (no abstract is necessary). Properly cite and reference any websites or documents you include to support the requirements of this assignment.Your cover page should contain the following: Title, Student’s name, University’s name, Course name, Course number, Professor’s name, and Date.
